Science or Science-y: Part I

When I entered an economics Ph.D. program at MIT in 2001 -- considered the best and most innovative department in the country -- I did so as a wide-eyed believer in the science of human behavior. Granted, like many young economists, I dismissed the "humans are rational" model that was still dominant at my undergraduate institution (the University of Chicago). But the exciting methods used by scholars such as Steve Levitt and Mark Duggan (whom I worked extensively with, as an undergraduate) presented a new frontier of social scientific research.
The mantra: "Let's not assume human behavior, rational or otherwise. Let's observe it."
What has happened since those years has been a rude awakening to the realities of social scientific research. The behavioral economics movement, which I deemed myself a part of, promised to scientifically model the vagaries of human decision-making into one grand theory of behavior. But in reality, the on-the-ground research was hopelessly piecemeal. Behavioral economics was grafted, like a zombie appendage, onto the dominant "rational choice" model. And it seemed lost, as an actual description of human decision-making processes. More importantly, field experiments began to appear showing that many of the results obtained in the behavioral economics "laboratory" (i.e., surveys of college undergraduates) could not be replicated in the real world.
I lost enthusiasm for the discipline, as a result of this intellectual whiplash (and ended up, like so many other lost souls, in law school). But one thing that I did learn from that time: even for the best and most well-trained researchers, social science is hard. Very, very hard. And when someone tries to make it sound easy -- when they simplify something as complicated as human social behavior into precise numbers and deterministic models -- they are probably pulling the (vegan) wool over your eyes.
It is with this experience that I discuss the recent spate of "scientific" claims relating to animal activism (and a recent article by Nick Cooney - Changing Vegan Advocacy from an Art to a Science.)
Some examples:
- "or every 100 leaflets you distribute on a college campus, you’ll spare, by a conservative calculation, a minimum of 50 animals a year a lifetime of misery. That’s one animal spared for every two leaflets you distribute!" (from Farm Sanctuary)
- "In summary, the data suggests that among college students Something Better booklets spare at least 35% (and probably closer to 50-100%) more animals than Compassionate Choices booklets." (from the Humane League)
- "THL switched their entire ad campaign to the more effective video. In doing so, they probably made their campaign about 70% more effective. And that means that if they reach the exact same number of people, they will go from sparing 217,000 animals last year to sparing 369,000 this year." (from "Changing Vegan Advocacy from an Art to a Science")
Conclusions such as these have the veneer of scientific respectability. They involve "experiments" and "testing" and give us startlingly exact numbers ("369,000 animals spared! 35% more effective!"). But the truth is that these studies are not "Science."
They are "Science-y" -- claims that clothe themselves in the language of scientific rigor without having anything of substance, underneath them.
This post is the first of a multi-part series that explains why.
1. Controls
Perhaps the fundamental problem, in making social science a "science," is that we cannot easily do controlled experiments. In chemistry, we can be confident that every sodium atom is the same as the last. So when we combine sodium with one reagent, in Trial A, and another reagent, in Trial B, we can be confident that any difference in the trials is due to the differences in the two reagents (rather than a difference in the sodium atoms, which are all the same.)
This is not true, when we are talking about separate human beings. Every one of us is different. This makes "controlled experiments" extraordinarily difficult. If I give Jane leaflet X, and John leaflet Y, and Jane responds while John does not, that could be because leaflet X is a better leaflet. But it could also be because John is a different person from Jane, and not very receptive to leaflets of any type. Indeed, leaflet Y -- the leaflet that did not cause a response in John -- might very well be better than leaflet X, despite our data. If we had given leaflet Y to Jane, perhaps she would have changed to an even greater extent than she changed with leaflet X. And yet our data, misleadingly, suggests the opposite!
Scientists use many different methods to get around this problem, but they all go under the umbrella of "experimental controls." And the basic idea is the same: to limit (or otherwise account for) the moving parts in a study in a way that makes clear that only the variable we care about (e.g. the difference between two leaflets, in the above example, or the differing regents, in the experiment with sodium) could be causing any differences in the results.
Upshot: If you see a study that does not even make an attempt at experimental controls -- specifically look for the word "control" -- you should be immediately skeptical.
2. Endogeneity
Most studies performed by trained researchers will make an attempt at experimental controls. However, there is a related and far more difficult issue, in social science, that even trained economists and statisticians struggle with. It's called "the endogeneity problem."
The basic question of "endogeneity," in layman's terms, is this: Suppose we see a shift in a pattern of data. How do we know that shift is a result of causality, rather than mere correlation? And, even if there is causality, how do we know that the shift in the data is caused by our experiment's external ("exogenous") influence, rather than an internal ("endogenous") influence that we are ignoring?
Let's use a concrete example to illustrate. Suppose that we have data showing that 2% of people who take a leaflet, at the University of Chicago, became vegetarian, compared to 1% of those in a control group.
Powerful evidence of the impact of leafleting, you say? (After all, the rate of vegetarianism seemingly doubled for those who took a leaflet!)
Absolutely not. Because we have not ruled out an alternative causal mechanism -- namely, that people who are already inclined toward vegetarianism are, because of that inclination, more interested in vegetarian leaflets.
And, in fact, that alternative explanation seems quite compelling. If people are already thinking about vegetarianism, surely they would be more interested in a vegetarian leaflet! But if we just look at the raw data, we can't distinguish between this theory and the original hypothesis. The data is exactly the same, for two very different models of the world.
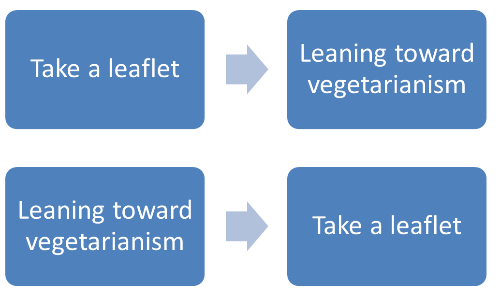
This phenomenon -- reverse causality -- is one of the notable examples of endogeneity.
That's not the end of the story, however, because even in cases where we can rule out reverse causality -- perhaps because there's no plausible story as to how causality could run both ways -- there is still an even larger problem: omitted variable bias.
Take, again, the example we discuss above, where 2% of students who take a vegetarian leaflet go vegetarian (compared to 1% of the control). Say that we have ruled out reverse causality by determining that, contrary to our intuition, vegetarian-leaning students are not more likely to take vegetarian leaflets. (Assume, contrary to fact, that there are multiple peer-reviewed studies showing that vegetarian-leaning students feel that they don't need a vegetarian leaflet, and are thus no more likely to take a leaflet than average.) Then, if we see an association between leafleting and vegetarianism, can we say with confidence that the vegetarianism was caused by the leaflet?
Again, the answer is resoundingly, "Absolutely not!" Because we have not ruled out omitted variables that could be causing students to BOTH take a leaflet, AND be inclined toward vegetarianism. For example, suppose that Dennis Kucinich, the famous vegan Congressman, had given a talk about veganism a few months prior to our leafleting study. And suppose that Dennis's powerful words caused many people to both: be more inclined to take a vegetarian leaflet; AND be more inclined toward vegetarianism. Indeed, if Dennis were actually a persuasive speaker, we would expect both effects from his speech.

Data drawn from the above "Dennis Kucinich" model will show that taking a leaflet is associated with vegetarianism. But, in actual effect, the leaflet has not caused the vegetarianism. Rather, under this model, it is the Dennis Kucinich speech that caused both the taking of the leaflet AND the vegetarianism. The association between those last two variables -- the leaflet and vegetarianism -- would simply be a statistical artifact!
Upshot: if a study does not even make an attempt to deal with "endogeneity" -- and, in particular, reverse causality and omitted variable bias -- you should be immediately skeptical.
3. Observer Bias
People have a tendency to interpret information in a way that confirms their pre-existing beliefs. This explains why, for example, a Bulls fan and a Knicks fan, can watch the exact same basketball replay, and come to very different conclusions as to whether Michael Jordan committed a foul. The term for this is "confirmation bias," but there is a broad cluster of related phenomena -- reporting bias, publication bias, attentional bias -- that fall under the same general umbrella:
People, even the best of scientists, see what they want to see.
This effect is especially important, when we are talking about an area where small differences in observation lead to big effects in our ultimate conclusions. Let me illustrate. Suppose again that we are doing a leafleting study at the University of Chicago, and 10 out of 100 data points are ambiguous in some way. Perhaps these data points are poorly marked, and require interpretation by the researcher in determining how the respondent actually responded to a survey question.
If a researcher is merely 1.1 times more likely to conclude that these ambiguous data points "confirm the hypothesis" over someone who is truly objective, that would lead to a 1% difference in the final results -- that is, the same doubling of vegetarianism that we previously saw! But the entire effect would be the result of observer bias, and not any real effect of the leaflet. (In reality, observer bias is probably far more than 1.1x in interpreting ambiguous data. Consider how much more likely a Knicks fan is to call a foul on Michael Jordan.)
It's even worse than this, however, because even if researchers are scrupulous in only hiring neutral data collectors, or engage in blind trials, there is still a fundamental issue of reporting bias. Studies that lead to results that do not favor the researcher's desired outcome are ignored. (It is not interesting, after all, to publish a study with no results!) Studies that do show such results, in contrast, are trumpeted. But this is no different from a child who rolls a die multiple times, finally gets a "6", and concludes to the world, "This die only has 6's."
If you do a study enough times, you will eventually get the result you want, particularly when the natural variance in the study -- i.e. the randomness in outcomes that has nothing to do with any variable of interest -- is significant compared to the expected effect of the intervention!
This has nothing to do with active deception, of course (though actual fraud is also quite common). It could very well be that the researchers genuinely believe that the study's results are valid. But until we have independent replication of the results -- ideally by trained and peer-reviewed researchers -- we should be very wary of drawing hasty conclusions. And when we see an organization regularly and conveniently finding "data" in support of its own methods and materials, over other organizations' (see, for example: the Humane League Labs' recommendations, which hugely favor their own materials, even over seemingly similar materials from rival organizations), or privileging one approach, based on a single study, while ignoring a large body of literature leading to the exact opposite conclusion, we should be wary of their conclusions.
Upshot: if a study is not performed by an independent researcher, OR if a study has not been replicated by independent researchers, you should be immediately skeptical.
4. Summing up
The animals rights movement has not seen nearly as much success as most of us would like. Temporary blips in meat consumption are trumpeted as major victories, even as those gains are lost in a year or less. (I'll be blogging about this soon; stay tuned.) Reformist laws are celebrated, after years of passionate efforts, only to be repealed or ignored by the powers that be. And through it all, the tide of increasing violence grows and grows, especially in the developing world.
In this context, it is easy to grasp for seemingly "rigorous" and "scientific" conclusions. Frightening things are happening, and uncertainty, as Harvard's Daniel Gilbert has written, is an emotional amplifier. But "science" is not an easily-obtained conclusion; it is an arduous and failure-filled process. And before we hastily jump towards accepting any particular conclusion as "science", we should ask ourselves these important questions about process:
- Is there a problem with experimental controls?
- Is there an endogeneity issue?
- Is the observer biased?
If we answer any of these questions, to the affirmative, then the study is not valid Science. It's just Science-y. And we should be highly skeptical of its results.
(In Part II and Part III of this series, I will discuss the so-called "small N" problem, and the scientific importance of asking the right question. Stay tuned! Also, be sure to check out DxE's open meeting this weekend, on the subject of Measuring Progress, with two great speakers -- and professional data scientists -- Allison Smith and David Chudzicki.)